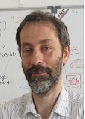
Siniša Šegvić
University of Zagreb, Croatia
Title: Pixel-level image understanding for smart and autonomous vehicles
Biography
Biography: Siniša Šegvić
Abstract
Semantic segmentation performs pixel-level image understanding by associating each image pixel with a meaningful class such as 'road', 'terrain', 'sidewalk' or 'person'. The resulting semantic map reveals the kind of surface terrain in front of the vehicle, and may be used to recover the traversability map required for motion planning. This capability makes semantic segmentation one of t he most important computer vision tasks in the automotive context. Today, state of the art semantic segmentation results are obtained with deep end-to-end trained convolutional models. However, direct application of popular and well understood image classification architectures would lead to poor semantic segmentation performance. The main obstacles are large variation of object scale, and strict memory limitations of contemporary GPUs. Recent works overcome these obstacles by careful architectural adaptations. As a result high semantic segmentation accuracy today can be achieved on large images in real-time, while more training data would likely further improve the results. Specifications of upcoming embedded hardware platforms promise low-power real-time onboardope ration and provide directions for exciting real-world applications.